Keynote Speaker
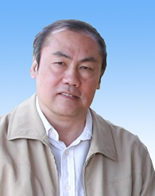
Qin Zhang
Professor, Institute of Nuclear and New Energy Technology and Department of Computer Science and Technology, Tsinghua University, ChinaSpeech Title: Trustworthy AI Aiding Clinical Diagnosis in Real World
Abstract: Medical AI needs to be trustable. However, the current AI such as Deep Learning and Large Language Models is black-box without explainability. DUCG (Dynamic Uncertain Causality Graph) is a newly developed trustworthy medical AI for clinical diagnosis in general practice and beyond. It graphically represents the medical knowledge and makes probabilistic reasoning with explainability, transparency and inherent invariance in different application scenarios. It is causality-driven instead of data-driven, so that it does not have problems such as data collecting, labeling, training, hallucination, generalization, privacy, bias, high cost and high energy consumption, etc. Cooperated with clinical experts deeply, 46 chief complaint models covering more than 1,500 diseases have been constructed and applied in the real-world in China. 41 models are retrospectively verified by third-party grade IIIA (the highest grade in China) hospitals. The verified diagnostic precisions of the 41 models are no less than 95%, in which the precision for every disease including the uncommon one is no less than 80%. More than 2 million real diagnosis cases have been performed, in which the prospective study was executed to some extent. So far, 17 cases have been identified as incorrect. The mistakes in DUCG were traced and corrected. These mistakes have not been reported since the corrections. Statistics show that DUCG can improve the ability of general practitioners to diagnose diseases several times more than without DUCG. The chief complaints are:
dizziness, headache, nasal congestion (including runny nose), nosebleeds, sore throat (including dry throat, itching, and foreign body sensation), jaundice, swallowing difficulties, cyanosis, coughing and sputum production, difficulty breathing (including chest tightness and shortness of breath), neck and back pain, hemoptysis, lymphadenopathy, chest pain, palpitations, vomiting blood, joint pain, nausea and vomiting, menstrual reduction or amenorrhea, limb numbness, edema, bloody stools, constipation, vulvar itching, abnormal vaginal secretions, vaginal bleeding, rash, fever, anemia, obesity, weight loss, children's fever, abdominal pain, diarrhea, fainting. bloating, oliguria or anuria, lower urinary tract symptoms (frequent urination, urgency, painful urination, gross hematuria, difficulty urinating, polyuria, leakage), lumps A diagnostic model was constructed and tested for more than 70 chief complaint symptoms, including acid reflux, heartburn, belching, ear symptoms (including ear pain, tinnitus, and deafness), scrotal pain, insomnia (including difficulty falling asleep, easy awakening, and early awakening), eye pain, redness, and tearing (including eye pain, redness, tearing, dryness, itching, swelling, and foreign body sensation), visual dysfunction (including visual abnormalities, visual field defects, visual distortion, color vision changes, flash sensation, diplopia, strabismus, and floaters), toothache.
Key Words: Trustworthy, Causality, Diagnosis, Explainability, Probabilistic Reasoning.
Reference:
Zhan Zhang, Qin Zhang, et al. Methodology and real-world applications of dynamic uncertain causality graph for clinical diagnosis with explainability and invariance, Artificial Intelligence Review, (2024) 57:151, DOI: 10.1007/s10462-024-10763-w.
Biography: Qin Zhang graduated from Tsinghua University, Beijing, China, with BS., MS. and Ph.D. Degrees in nuclear engineering in 1982, 1984 and 1989 respectively. He was a visiting scholar with University of Tennessee, Knoxville, TN, USA, and University of California, Los Angeles, CA, USA, from 1987 to1989, working on system reliability engineering and intelligent fault diagnoses. He is a professor of Institute of Nuclear and New Energy Technology and Department of Computer Science and Technology, Tsinghua University, emeritus member of China Association for Science and Technology, member of International Nuclear Energy Academy, fellow of China Association for Artificial Intelligence (CAAI) and director of the specialized committee for causality and uncertainty in AI of CAAI, consultant of the specialized committee for wise medical care of CAAI, Chief scientist of Beijing Yutong Intelligence Technology corp., ltd. He is the former vice president of China Association for Science and Technology, and the standing member of the 13th CPPCC. He originally developed a new AI model called Dynamic Uncertain Causality Graph for fault diagnoses and disease diagnoses. The DUCG medical system has been applied in 4 districts and a county in China for a few years, covering 46 chief complaints such as headache, dizziness, joint pain, etc., including 1500 diseases. More than 2 million cases have been diagnosed with only 17 mistakes identified. All the mistakes were traced and corrected without further mistake reports about them.